The recent advancements in OpenAI's Q* algorithm speculated to be a hybrid of Q learning and AAR (Adaptive Agent Reasoning), represent a significant leap towards achieving Artificial General Intelligence (AGI). This breakthrough, while raising concerns about its potential impact on humanity, marks a pivotal moment in the journey of AI evolution.
Coming from the recent OpenAI debacle, it seems that researchers recently warned their board about an AI breakthrough called Q* that could "threaten humanity." While details remain scarce, analyzing the potential meanings behind Q* provides intriguing clues into OpenAI's latest innovations in goal-oriented reasoning and search algorithms. If realized fully, Q* may represent a pivotal step towards more general artificial intelligence.
What Could Q* Signify?
Current speculation centres on Q* representing an integration between two key algorithms - Q-learning and A* search. Q-learning provides a framework for an AI agent to learn which actions maximize "rewards" that bring it closer to a defined goal state. The A* ("A-star") algorithm enables efficient searching through large spaces of potential routes to find the optimal path to a goal.
Together, these algorithms could significantly improve an AI's ability to reason about and pursue complex, long-term objectives - deciding on optimal choices and strategies to navigate barriers and setbacks. Rather than just excelling at narrow tasks, Q* may allow an AI to chain tactical decisions towards accomplishments.
Let's dive deeper.
Understanding Q*: A Hybrid of Learning and Reasoning
The Essence of Q-Learning
At its core, Q-learning is a reinforcement learning algorithm that empowers AI agents to make optimal decisions in varying states. It forms the foundations for teaching an artificial intelligence how to dynamically interact with and adapt to its environment in order to maximize cumulative "rewards" that bring it closer to its goals.
The agent starts by taking various actions and observing how these affect the environment - such as moving directions in a grid or applying different mathematical operations to balance an equation. Each action earns a numeric reward, whether positive or negative. Over time, the agent learns to associate the rewards with state-action pairs to build a quality matrix or "Q" table mapping out which choices work best for reaching the objective from any given situation.
This cycle of trial-and-error and reward-based feedback teaches the AI how its decisions influence outcomes. Just as humans build intuition and heuristics from experience about which strategies work well under which circumstances, so too can a Q-learning algorithm develop expertise in making choices best calibrated to efficiently achieve its defined goals.
Adaptive Agent Reasoning (AAR)
The A* or "A-star" search algorithm provides an excellent complement to Q-learning in enabling more robust agent reasoning. While Q-learning focuses on determining optimal actions from different states, A* deals with efficiently navigating or traversing through large problem spaces to reach goal states - whether in a physical environment or more conceptual scenario.
Just as humans leverage memory and environmental cues for wayfinding, A* allows an agent to leverage its growing knowledge to judiciously guide exploration. It reaches beyond simplistic brute force approaches of exhaustively trying every possible route blindly. The algorithm develops heuristic cost estimates to focus its search efforts on the most promising nodes and paths first - pruning away clearly suboptimal branches.
As the agent maps out the problem terrain, finding barriers and breakthroughs, A* helps it bypass dead ends and detours. Rather than starting its reasoning from scratch when faced with obstacles, the agent can incorporate accumulated insights on the geometry of the solution space to adapt its approach and identify more optimal possibilities forward. This type of dynamic, lifelong learning of the environment's layout enables more targeted, effective navigation towards accomplishing objectives.
Together with Q-learning's ability to associate actions with rewards, AAR enables agents to shape intelligent, strategic processes for accomplishing goals - getting from Point A to Point B, whether traversing a grid or balancing an equation. Just as humans leverage experience and environmental cues to pursue objectives more efficiently, combining learning algorithms allows AI to better emulate such goal-oriented reasoning.
Q* as a Fusion of Q-Learning and AAR
The speculation that Q* represents an integration between Q-learning and the A* search algorithm is intriguing. This hybridization would suggest that Q* enables AI agents to not only learn optimal decisions from environmental feedback, but also efficiently navigate through the complex problem-solving space needed to accomplish multi-step tasks.
Together, Q-learning and AAR can cover complementary facets of intentional behavior - determining the best actions for progress as well as intelligently planning the paths to enact those actions across situations to reach goals. Blending these algorithms could significantly enhance how artificial agents emulate the human capability to construct and execute complex plans.
Just as people leverage intuition from experience about both productive strategies and wayfinding when pursuing objectives, Q* has the potential to accumulate insights over time about both as well. This more well-rounded learning and reasoning could empower AI systems to self-determine robust processes for accomplishing goals - whether solving math problems or broader challenges like crossing the ocean without traditional means of transport.
If future investigation confirms that OpenAI has achieved this blend of sophisticated learning architectures in Q*, it would provide a powerful demonstration of AI with more expansive capacities for goal-oriented reasoning and behavior. Given animal and human cognition alike evolved such flexible pursuit of self-determined goals as cornerstones of intelligence, Q* may offer a glimpse towards more human-aligned AI.
Speculations and Implications
Unprecedented Accuracy in Problem Solving
One of the most intriguing reports about the early Q* results claims the algorithm was able to solve math problems with 100% accuracy - a level of precision not previously achieved by other AI systems. At surface level, flawless math performance may seem trivial. However, such flawless execution likely signifies something much more profound regarding Q*'s underlying reasoning processes.
For an AI to calculate solutions or balance equations reliably correct, it cannot simply pattern match or mechanically manipulate numbers and symbols based on rote rules. Those approaches still risk blind spots or brittleness. Instead, solving math problems well requires deeply analyzing the abstract structures and relationships within each problem to decode the suitable techniques to apply. It demands fluid perception, interpretation and strategic goal-oriented thinking.
For Q* to perfectly select and execute the right sequential goal pursuit strategies to arrive at demonstrably correct solutions, it implies an unprecedented level of perceptual understanding and intentional analysis. Q* would need to not just broadly mimic human-like cognition but actually skillfully emulate particular facets of dynamic human reasoning, judgment and planning. Such versatile accuracy could enable AI to self-sufficiently conquer many more classes of problems - mathematical or otherwise.
This alleged feat underscores why Q* may be such a significant research breakthrough. More than just its end performance, the kind of robust and transferrable problem-solving capacity it demonstrates could greatly expand AI's real-world usefulness. And matching let alone exceeding human expertise in tasks requiring complex reasoning also suggests AI developing deeper comprehension, forethought and decision-making proficiency as well - getting us closer than ever to more human-aligned artificial general intelligence.
A Cognitive Neuroscience Perspective
Analyzing a breakthrough like Q* from a cognitive neuroscience view provides further intrigue. The speculated integration between reinforcement learning and search algorithms closely parallels mechanisms in the human brain for goal-oriented reasoning and cognitive control. This resemblance suggests Q* development may be equipping AI models with an intrinsic capacity to purposefully navigate towards set objectives - closely emulating human decision-making.
Just as algorithms track rewards and state values to optimize progress, humans encode perception and feedback within structures like the prefrontal cortex to update internal representations of goal proximity and strategy effectiveness. Likewise, the hypothesized tree search processes in Q* for dynamic planning alignment with regions like the parietal cortex used to navigate abstraction in the mind and external spaces.
Moreover, subconscious emotions in people play integral roles for flexible goal pursuit - with frustration signaling obstructed progress. If further advances in Q* explore integrating similar heuristic processes and affective signals rather than pure logic, it would only strengthen ties to human-like cognition.
At core, the postulated inner workings of Q* closely mirror neurocognitive architectures for strategic planning, redirection, abstraction and intentionality. So while full confirmation remains needed on the exact nature of this AI breakthrough, viewed through a neuroscience lens, it suggests perhaps the closest ever computational approximation of deliberate goal-driven human thinking.
The Threat to Humanity: A Real Concern?
The dire language from OpenAI researchers warning of Q* potentially threatening humanity should certainly give us pause. While the broader context around these reported statements remains limited, it spotlights important ethical considerations as AI capabilities expand. Does a breakthrough like Q* genuinely put us existentially at risk?
There are real tensions in balancing transformative upsides and downsides from more generally intelligent goal-driven algorithms. The same advances allowing AI to cure diseases, boost prosperity or expand knowledge could also empower catastrophic harm if misdirected either intentionally or accidentally. We must thoughtfully shape development pathways to amplify benefits and control hazards.
But while sobering to consider worst case AI scenarios, this tension between promise and peril has parallels throughout human innovation history - from nuclear chain reactions to genetic engineering. With diligent, democratic oversight and responsible innovation cultures, we can work to reap AI progress's benefits while addressing the complex safety challenges that understandably give technologists and philosophers alike pause in the development race.
For now, while Q* hints at exciting possibilities in AI reasoning, we lack clarity to definitively conclude it enables full autonomous agency or transcends alignment with human values. Wise voices urge proactive consideration of risk scenarios, but we should be wary of alarmist hyperbole. If indeed representing a breakthrough, OpenAI along with policymakers have an obligation to transparently ensure this and future advances develop cautiously and concertedly with societal interests in mind. The outcomes remain in human hands.
Why Math Tests Matter More Than They Seem
Initial reports suggest OpenAI tested Q* on math problems - achieving 100% accuracy where other AIs still struggle. On a surface level, this seems minor, but it hints at something deeper. Solving math problems doesn't just require numerical manipulation skills - it needs strategic thinking to decode the problem structure and identify suitable techniques. If Q* performs well here, it shows potential to apply similar goal-oriented reasoning for making plans and decisions. Just as balancing an equation can require trying multiple approaches to reach the solution, real-world pursuits often demand fluid, adaptive strategy.
From Game Environments to the Real World
Reinforcement learning architectures frequently test AI agents in games and simulations first before attempting real-world applications. In navigating grids and game environments with clear boundaries, measurable rewards, and low stakes for mistakes, Q* would have an ideal space to learn and refine its goal-pursuit abilities.
If Q* proves capable of mastering complex objectives reliably in silico, the next step would be scaling to messier, unpredictable domains like robotics and business strategy. OpenAI likely recognizes both the breakthrough potential and risks here in taking the training wheels off advanced cognitive algorithms.
What's Next for AI Goal Reasoning
Q* represents just one point on the frontier of AI safety research around aligning smarter algorithms more closely with human values and ethics. As language models like GPT-3 and image generators like DALL-E 2 demonstrate expanded creativity, the need grows for control mechanisms so that powerful autonomous systems remain helpful assistants rather than potentially harmful adversaries.
If OpenAI has in fact achieved a leap forward with Q*, they surely have much work remaining to ensure this technology develops responsibly. But a solution that trains AI "assistants" to more reliably pursue and accomplish designated constructive, benign goals could be a very positive thing indeed. Both the breakthrough and uncertainty around Q* will be worth monitoring closely as we try to shape our AI future for the better.
References & Updates
I'll keep an ongoing mini-news feed on Q* here
Reuters reports
/cloudfront-us-east-2.images.arcpublishing.com/reuters/WRAGM7C3ONP4BO5NMF23ZGV2SA.jpg)
- 🚨 OpenAI researchers wrote to the board warning of a potentially dangerous AI discovery.
- 📃 The letter and AI algorithm were significant factors in CEO Sam Altman's ouster.
- 🤝 Over 700 employees threatened to quit in solidarity with Altman, possibly joining Microsoft.
- 🧠 The AI project, Q*, showed promise in artificial general intelligence, particularly in solving mathematical problems.
- 🕵️♂️ Reuters couldn't independently verify Q*'s capabilities as claimed by the researchers.
- 🛑 The letter raised concerns about AI's capabilities and potential dangers.
- 🤖 An "AI scientist" team at OpenAI was working on improving AI reasoning and scientific work.
- 🚀 Altman's leadership was pivotal in advancing ChatGPT and drawing significant investment from Microsoft.
- 🌐 Altman hinted at major advances in AI at a global summit before his dismissal.
OpenAI "Q" Paper
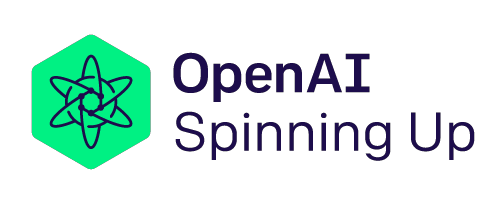
📘 Deep Deterministic Policy Gradient (DDPG) is an algorithm for environments with continuous action spaces.
🧠 DDPG combines learning a Q-function and a policy using the Bellman equation and off-policy data.
📈 The algorithm adapts Q-learning for continuous action spaces, using a gradient-based learning rule.
🤖 It includes two main components: learning a Q-function and learning a policy.
🎯 The Q-function learning uses mean-squared Bellman error, replay buffers, and target networks.
🔍 Policy learning involves finding the action that maximizes the Q-function through gradient ascent.
🔄 DDPG employs exploration and exploitation strategies, including adding noise to actions during training.
💻 The algorithm is implemented in both PyTorch and TensorFlow, with detailed documentation for each.
Other Q-related Papers
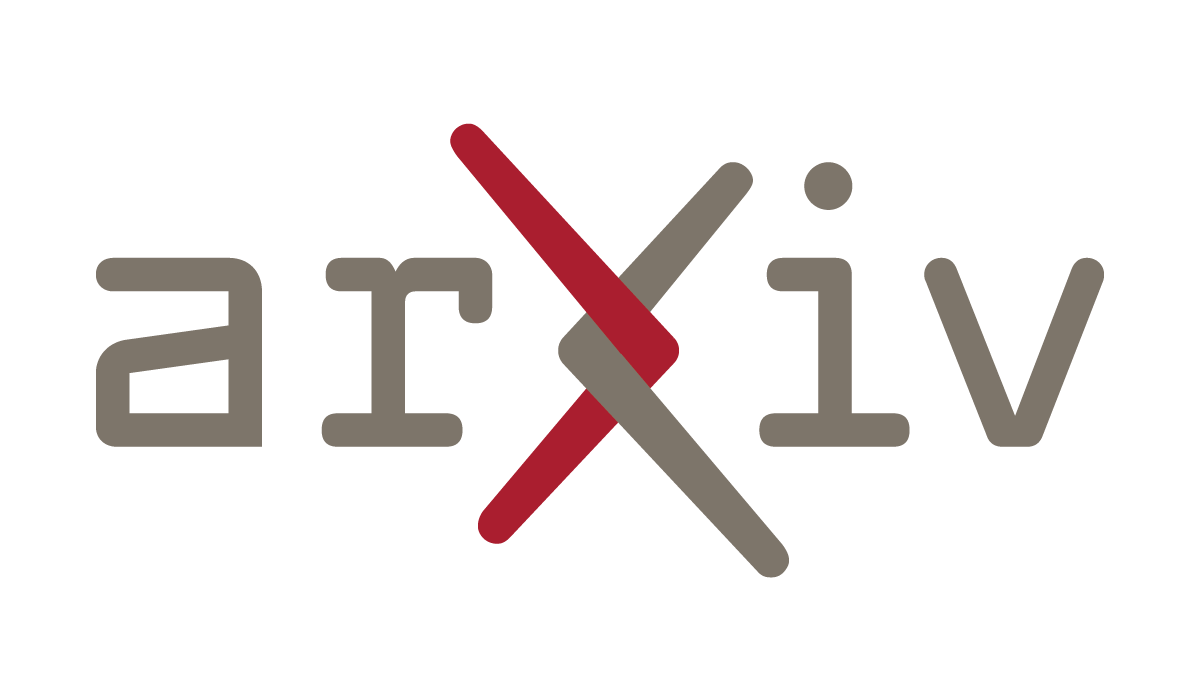
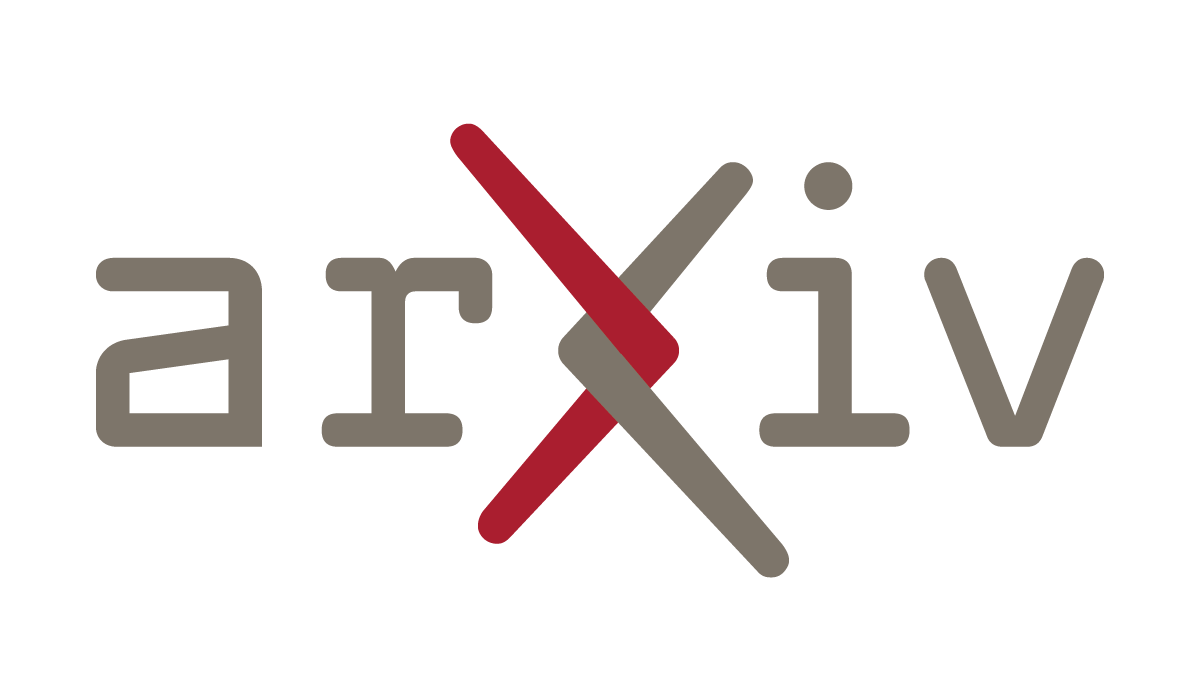
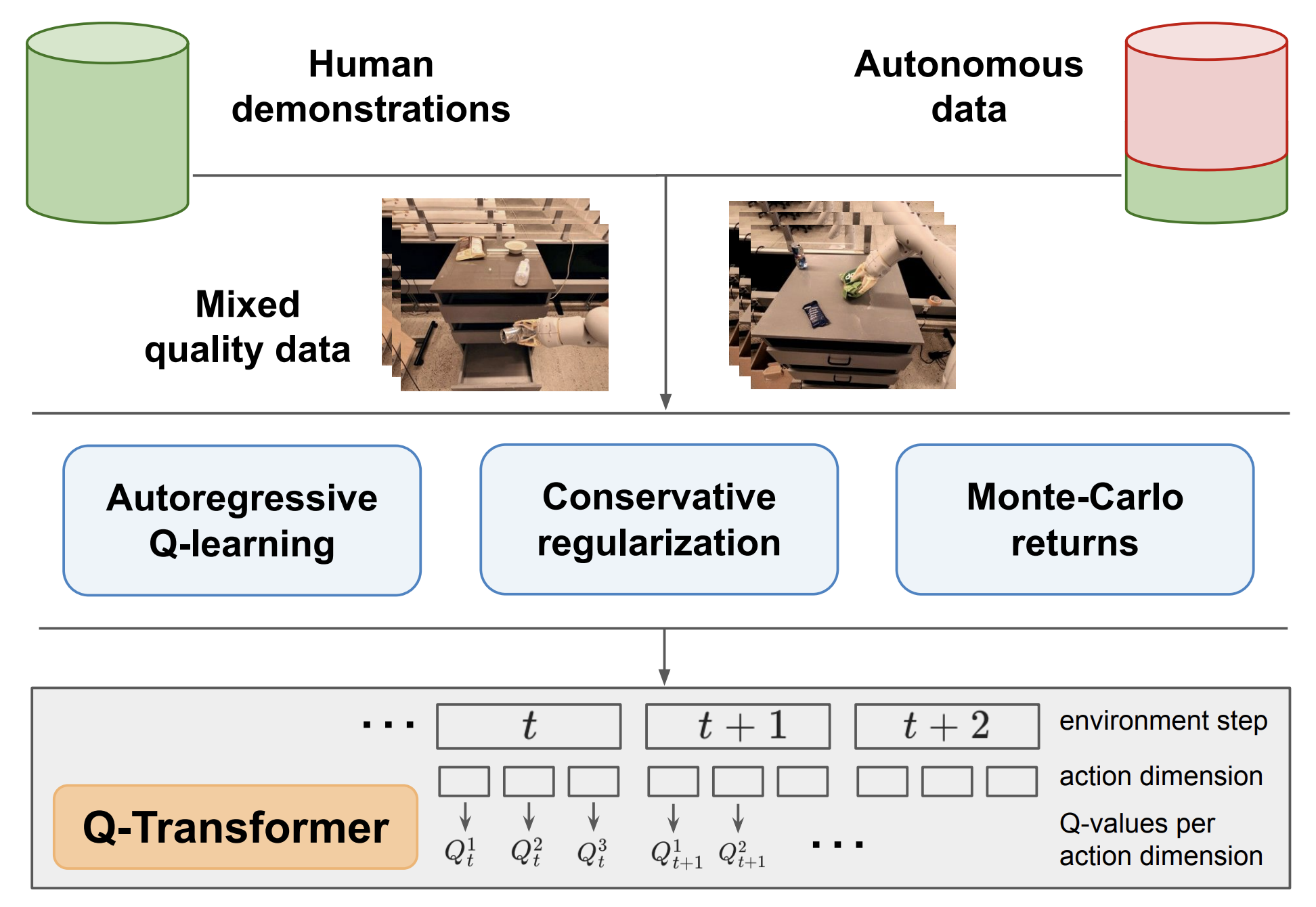

Ongoing Forum discussion
