In the rapidly evolving landscape of artificial intelligence, we are witnessing an unprecedented surge in the development and release of innovative AI-powered applications, such as ChatGPT, Midjourney, and Stable Diffusion. This AI revolution is transforming various industries, and the world of finance is no exception. Bloomberg, a global leader in financial technology, has recently joined the race with the release of a research paper on their specialized large language model for finance, dubbed BloombergGPT.
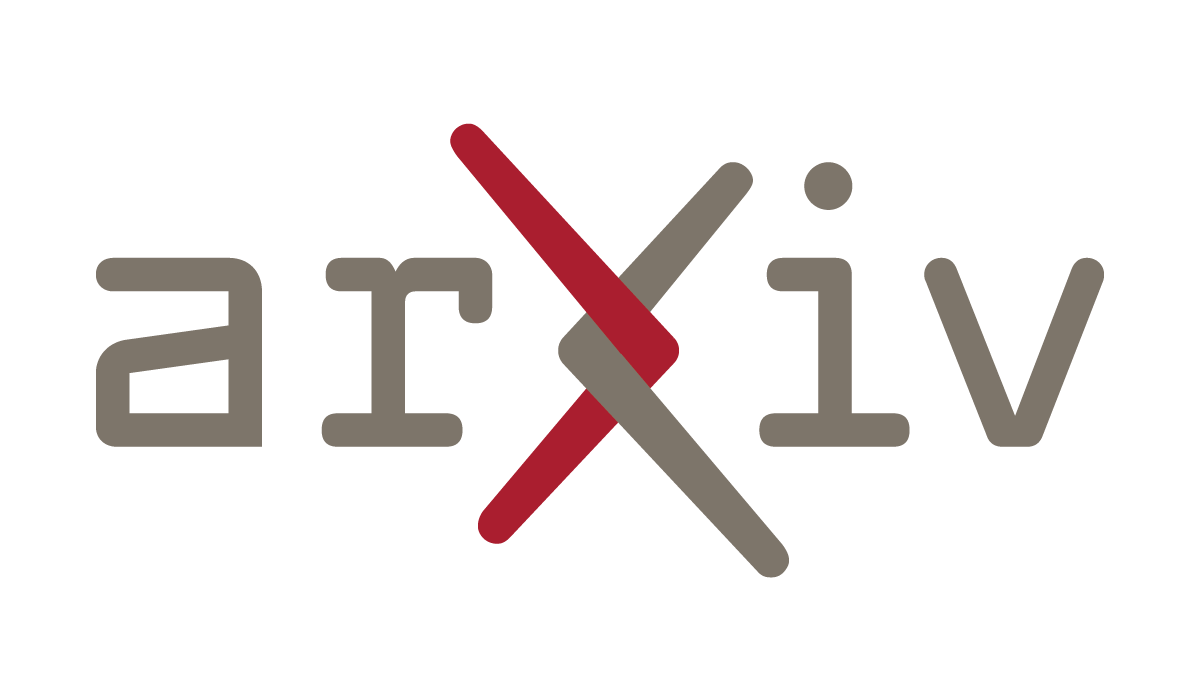
As the demand for more specialized and advanced language models continues to grow, BloombergGPT emerges as a trailblazer (sort of) in the financial sector, offering a unique solution tailored to the needs of the finance industry.
Understanding BloombergGPT
BloombergGPT is a 50 billion parameter language model specifically designed for the finance industry. It is smaller than GPT-4, but it claims to outperform existing models (GPT-3/GPT-4 were not included in the evaluation) without sacrificing performance on general language model benchmarks. Bloomberg has used a mix of financial and public data to train their model, with 50% of the data being financial and the other 50% being public.
BloombergGPT is the result of a mixed approach, combining general-purpose language models with domain-specific ones. While general models provide a solid foundation for various tasks, they cannot replace domain-specific models. The financial industry requires a specialized tool to handle its unique challenges.
To train BloombergGPT, Bloomberg leveraged its extensive archives of financial data, collected and curated over 40 years. These archives encompass a wide range of topics with careful tracking of sources and usage rights. By incorporating this data into public datasets, BloombergGPT's training corpus consisted of over 700 billion tokens.
Performance
BloombergGPT was compared with three other closely related models, GPT-NeoX, OPT66B, and BLOOM176B. These models were selected based on their model size, type of training data, overall performance, and accessibility. The comparison revealed that BloombergGPT demonstrated competitive performance across the board. Interestingly, although BLOOM176B is a much larger model, it is trained on data from more languages, making the comparison more balanced.
To measure the success of BloombergGPT, the model was validated on a range of benchmarks, including standard LLM benchmarks, open financial benchmarks, and a suite of Bloomberg-internal benchmarks designed to reflect real-world use cases.
Standard LLM Benchmarks
BloombergGPT's performance was first assessed using general LLM benchmarks, which test the model's capabilities across a broad range of tasks. The results showed that the mixed training approach taken with BloombergGPT allowed it to achieve performance on par with or better than existing general-purpose LLMs.
Open Financial Benchmarks
Next, BloombergGPT was tested on open financial benchmarks to gauge its effectiveness within the financial domain. Here, the model significantly outperformed its counterparts as it demonstrated a deep understanding of the complexities and intricacies of the financial industry.
Bloomberg-Internal Benchmarks
Lastly, the model was validated using Bloomberg-internal benchmarks specifically designed to reflect real-world use cases within the financial domain. These benchmarks proved that BloombergGPT's mixed training approach led to a model that excelled in in-domain financial tasks, making it a powerful tool for the financial industry.
Absence of GPT-3 and GPT-4 in BloombergGPT Testing: A Cause for Concern?
It is noteworthy that BloombergGPT's performance was not benchmarked against GPT-3 or GPT-4, the current state-of-the-art language models. The absence of these prime models from the testing phase raises some important questions about the true capabilities of BloombergGPT.
Considering that GPT-3 and GPT-4 are widely recognized for their impressive performance and versatility, one would expect Bloomberg to compare their specialized financial model against these heavyweights to establish its credibility and superiority in the finance domain. Instead, Bloomberg opted to test their model against other models that, while impressive in their own right, do not perform at the same level as GPT-3 or GPT-4.
The reasons behind this decision are unclear. One possibility is that Bloomberg may have wanted to focus on comparisons with models that are more specialized in the financial domain, rather than general-purpose models like GPT-3 and GPT-4. However, this approach may be flawed, as GPT-3 and GPT-4 have shown remarkable capabilities in understanding and generating finance-related content as well.
Another possibility is that Bloomberg wanted to avoid direct comparisons with these top-tier models to prevent revealing potential shortcomings in their BloombergGPT's performance. By not benchmarking against GPT-3 and GPT-4, it may be easier for Bloomberg to claim significant improvements over other models without facing scrutiny over their model's true capabilities compared to the best in the field.
Without benchmarking against GPT-3 and GPT-4, it is difficult to gauge the true performance of BloombergGPT in the finance domain accurately. This could mean that BloombergGPT may require additional fine-tuning and training to be ready for production, as its performance relative to the top models remains uncertain.
Data Sets and Training
Bloomberg has used diverse structured and unstructured financial data sets for training their model. These data sets include company filings, financial websites, news sources, transcripts, Bloomberg News articles, opinions, and press releases. They claim that the use of these mixed data sets has helped their model achieve significant improvements over existing models.
FinPile: The Backbone of BloombergGPT
BloombergGPT's foundation lies in its comprehensive dataset called FinPile, which contains an array of English financial documents collected over the past two decades. The dataset is a rich blend of domain-specific and general-purpose text, ensuring that the model is well-rounded and capable of handling various financial tasks. Some noteworthy components of the dataset include news, filings, press releases, web-scraped financial documents, and social media content.
Financial Datasets: The Building Blocks of FinPile
FinPile is an amalgamation of different financial datasets that make up over 54% of the training data. These datasets provide BloombergGPT with a solid foundation in understanding the financial world. Let's explore the key components of FinPile in more detail.
Web Content (42.01% of training)
Bloomberg gathers web content from sites that contain financially relevant information. This collection, which forms the majority of FinPile, is classified primarily by the web domain's location. Unlike general-purpose web crawls, Bloomberg focuses on high-quality websites with financially relevant information, ensuring that the AI model is well-versed in its domain.
News Articles (5.31% of training)
News articles are a critical component of the financial world, providing insights and updates on market trends and company developments. FinPile includes news from a wide variety of sources, excluding those written by Bloomberg journalists. This dataset emphasizes reputable news sources relevant to the financial community, ensuring accuracy and minimizing bias.
Company Filings (2.04% of training)
Company filings are financial statements prepared by public companies and made available to the general public. These filings are dense with financial information and are essential for financial decision-making. Most filings in the dataset come from the SEC's online database, EDGAR. Bloomberg processes and normalizes these documents, making them an invaluable resource for the AI model.
Press Releases (1.21% of training)
Press releases are typically issued by companies to communicate financially relevant information. While similar to news stories in terms of content and style, press releases represent the majority of a company's public communications alongside filings.
Bloomberg Content (0.70% of training)
The Bloomberg content category consists of news articles and other documents authored by Bloomberg, such as opinions and analyses. This dataset focuses on content relevant to the financial community and covers a wide range of topics.
Features and Applications
BloombergGPT can be used for suggesting news headlines, assisting journalists, and answering finance-related queries. While these capabilities are also achievable by other large language models, BloombergGPT has one unique feature: it can generate Bloomberg Query Language (BQL), a proprietary language used by Bloomberg terminal users.
While this feature might be useful for Bloomberg terminal users, it raises the question of whether BloombergGPT is just another feature for their existing product or a true game-changer in the finance industry.
Some potential applications include for BloombergGPT are:
Sentiment Analysis
BloombergGPT's deep understanding of financial language allows it to accurately gauge sentiment in news articles, research reports, and social media posts. This can help investors and traders in making informed decisions based on market sentiment.
Named Entity Recognition
The model's ability to identify and categorize entities, such as company names, stock tickers, and financial instruments, can streamline the process of data extraction and analysis, enabling more efficient workflows for analysts and researchers.
Question Answering
BloombergGPT's proficiency in question answering can greatly improve the user experience for financial professionals. With its ability to understand complex queries and respond with relevant, accurate information, BloombergGPT can act as a powerful assistant in the world of finance.
Openness and Availability
Bloomberg's openness policy for their GPT is quite restricted. They do not share details about the model except for the generalities mentioned in their paper. This is because their core business revolves around providing access to data collected over decades, and they are wary of data leakage.
This raises concerns about the future of large language models created by for-profit organizations. Will they be open to the public, or will they remain exclusive features for their existing products?
The Future of Large Language Models in Finance
BloombergGPT is an interesting development in the world of large language models, particularly in the finance industry. However, its advantages over other general-purpose transformers are not entirely clear.
Moreover, the fact that BloombergGPT is not openly available and seems to be primarily a feature for Bloomberg terminal users suggests that it might not be as revolutionary as it initially appears.
As more companies develop their own large language models, it remains to be seen whether they will make their models available to the public or keep them behind paywalls as exclusive features for their products. This could significantly impact the potential benefits and accessibility of these models for the broader finance industry and beyond.
Optimizing the Finance World
BloombergGPT potential to revolutionize the way financial information is processed and analyzed has yet to be seen. Its ability to understand and generate content related to finance and economics means it can assist in tasks such as drafting reports, generating investment ideas, and even predicting market trends. The implications for the finance world are vast, with the possibility of increased efficiency and accuracy in decision-making, ultimately benefiting businesses and investors.
The Rise of Specialized Models
The success of BloombergGPT underscores the growing trend of specialized models in AI. As more industries recognize the value of AI in their specific niches, it is becoming increasingly clear that general-purpose AI models like OpenAI's GPT-3 are not always the most effective solution. Instead, tailored models designed to address specific industry needs can deliver more accurate and relevant results. In the case of BloombergGPT, the model's focus on finance and economics allows it to excel in its domain.
There is a significant debate in the AI research community about whether it is more advantageous to develop a new proprietary large language model (LLM) or to leverage existing, popular LLMs and enhance their performance through fine-tuning or embedding techniques. Each approach has its merits and drawbacks.
Developing a proprietary LLM allows for greater customization and control, potentially enabling a model that is specifically tailored to a particular domain, such as finance. This can result in improved performance and efficiency, as well as greater intellectual property rights for the organization that develops it. However, this process can be resource-intensive, time-consuming, and costly, particularly when considering the expertise and computational power required.
On the other hand, utilizing existing LLMs like GPT-3 or GPT-4 and fine-tuning or embedding them for specific domains can be more cost-effective and practical. These models have already demonstrated their versatility and ability to excel in various tasks. By fine-tuning or embedding domain-specific knowledge, researchers can capitalize on the strengths of these models while addressing their limitations. This approach, however, might not offer the same level of customization as developing a proprietary LLM and may also come with licensing fees or other costs associated with using popular models.
Ultimately, the choice between developing a proprietary LLM or fine-tuning existing models depends on the specific goals, resources, and requirements of the organization in question.
Navigating the Ethical Challenges
As AI models like BloombergGPT continue to advance, ethical considerations become increasingly important. Issues such as the potential for job displacement, data privacy, and the concentration of power in the hands of a few large corporations need to be carefully navigated. Ensuring that these technologies are developed and deployed responsibly will be crucial to maximizing their benefits while minimizing their potential harm.
Opinion & Conclusion
BloombergGPT is a noteworthy development in the large language model space, especially for the finance industry. However, its actual advantages over other general-purpose transformers are not entirely evident, and its limited openness raises concerns about the future of large language models created by for-profit organizations.
While it is too early to determine the long-term impact of BloombergGPT, it will be interesting to see how other companies approach the development of their own large language models and whether they choose to make them openly available or restrict access as Bloomberg has done.